AI: Detecting and Avoiding Customer Churn is Critical
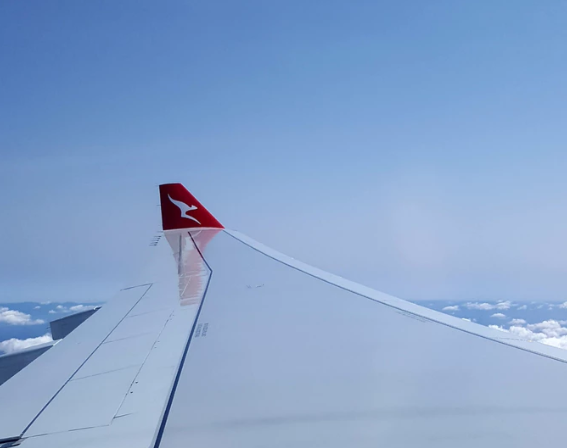
I’ve flown a lot over the years. What continues to strike me though, is how poorly airlines use machine learning and AI, even when they are in strong competitive environments. A key indicator is detecting and avoiding customer churn. Let me give you an example:
We flew with QANTAS and with their partners in One World for many years. We were both platinum and I’d been platinum for many years. At a recent peak a few years ago, we were flying once or twice a week. That’s not a crazy amount, but it’s enough. And it’s certainly enough to be able to see a purchasing pattern.
We finally got pretty fed up some years back, and one February, we said “enough”, and stopped flying with them.
But what fascinated me was how the airline reacted to that.
At the end of the year, I got an email pointing out they’d noticed that I didn’t fly as much that year, but because of previous custom, they’d keep my status in place.
After another whole year of not flying, I got another email saying they’d noticed a drop in my custom, and that they’d have to drop me down to Gold. Same thing again the next year.
But at no stage did they ever seek to work out what went wrong.
When could they have actually detected the change? Probably a month or two after we stopped. At that point, there’s always a chance you can recover the situation. Every business teacher will tell you how much harder it is to gain a customer than to avoid losing them in the first place, and how very much harder it is to regain a lost customer.
Look at your own businesses, and ask yourself if you have systems in place to detect changes in your customers’ behaviors, particularly if they’ve stopped dealing with you. Don’t just detect total volumes over each year. Look for changes in behavior.
2018-10-12